Analisis descriptivo multivariado.#
Universidad Central#
Maestría en analítica de datos#
Métodos estadísticos para analítica de datos.#
Docente: Luis Andrés Campos Maldonado.#
import warnings
warnings.filterwarnings("ignore")
import pandas as pd
import numpy as np
import matplotlib.pyplot as plt
import seaborn as sns
import plotly.express as px
##
sns.set_theme()
Cereal#
Vamos a cargar la base de datos de Cereal, que contiene información nutricional de diferentes marcas de cereales.
url = "https://raw.githubusercontent.com/lacamposm/Metodos_Estadisticos/main/data/Cereal.csv"
df = pd.read_csv(url, sep=";", decimal=",", index_col=0)
df.head()
Fabrica | Calorias | Proteina | Grasa | Sodio | Fibra | Carbohidratos | Azucares | Potasio | Vitaminas | |
---|---|---|---|---|---|---|---|---|---|---|
Cereal | ||||||||||
100% Bran | N | 212.12121 | 12.121212 | 3.030303 | 393.93939 | 30.303030 | 15.15152 | 18.181818 | 848.48485 | enriched |
All-Bran | K | 212.12121 | 12.121212 | 3.030303 | 787.87879 | 27.272727 | 21.21212 | 15.151515 | 969.69697 | enriched |
All-Bran with Extra Fiber | K | 100.00000 | 8.000000 | 0.000000 | 280.00000 | 28.000000 | 16.00000 | 0.000000 | 660.00000 | enriched |
Apple Cinnamon Cheerios | G | 146.66667 | 2.666667 | 2.666667 | 240.00000 | 2.000000 | 14.00000 | 13.333333 | 93.33333 | enriched |
Apple Jacks | K | 110.00000 | 2.000000 | 0.000000 | 125.00000 | 1.000000 | 11.00000 | 14.000000 | 30.00000 | enriched |
Observamos los tipos de datos en cada una de las columna del DataFrame.
df.dtypes
Fabrica object
Calorias float64
Proteina float64
Grasa float64
Sodio float64
Fibra float64
Carbohidratos float64
Azucares float64
Potasio float64
Vitaminas object
dtype: object
Con el método select_dtypes()
podemos obtener las columnas cuyos valores son numéricos.
df_numeric = df.select_dtypes(np.number)
df_numeric
Calorias | Proteina | Grasa | Sodio | Fibra | Carbohidratos | Azucares | Potasio | |
---|---|---|---|---|---|---|---|---|
Cereal | ||||||||
100% Bran | 212.12121 | 12.121212 | 3.030303 | 393.93939 | 30.303030 | 15.15152 | 18.181818 | 848.48485 |
All-Bran | 212.12121 | 12.121212 | 3.030303 | 787.87879 | 27.272727 | 21.21212 | 15.151515 | 969.69697 |
All-Bran with Extra Fiber | 100.00000 | 8.000000 | 0.000000 | 280.00000 | 28.000000 | 16.00000 | 0.000000 | 660.00000 |
Apple Cinnamon Cheerios | 146.66667 | 2.666667 | 2.666667 | 240.00000 | 2.000000 | 14.00000 | 13.333333 | 93.33333 |
Apple Jacks | 110.00000 | 2.000000 | 0.000000 | 125.00000 | 1.000000 | 11.00000 | 14.000000 | 30.00000 |
... | ... | ... | ... | ... | ... | ... | ... | ... |
Triples | 146.66667 | 2.666667 | 1.333333 | 333.33333 | 0.000000 | 28.00000 | 4.000000 | 80.00000 |
Trix | 110.00000 | 1.000000 | 1.000000 | 140.00000 | 0.000000 | 13.00000 | 12.000000 | 25.00000 |
Wheat Chex | 149.25373 | 4.477612 | 1.492537 | 343.28358 | 4.477612 | 25.37313 | 4.477612 | 171.64179 |
Wheaties | 100.00000 | 3.000000 | 1.000000 | 200.00000 | 3.000000 | 17.00000 | 3.000000 | 110.00000 |
Wheaties Honey Gold | 146.66667 | 2.666667 | 1.333333 | 266.66667 | 1.333333 | 21.33333 | 10.666667 | 80.00000 |
65 rows × 8 columns
## Así mismo con las columnas de tipo "object"
df_object = df.select_dtypes(include=["object"])
df_object
Fabrica | Vitaminas | |
---|---|---|
Cereal | ||
100% Bran | N | enriched |
All-Bran | K | enriched |
All-Bran with Extra Fiber | K | enriched |
Apple Cinnamon Cheerios | G | enriched |
Apple Jacks | K | enriched |
... | ... | ... |
Triples | G | enriched |
Trix | G | enriched |
Wheat Chex | R | enriched |
Wheaties | G | enriched |
Wheaties Honey Gold | G | enriched |
65 rows × 2 columns
Vamos a recoger en un lista los nombres de las columnas que son de tipo “numerico”.
Ejercicio 1.#
En un lista ubique todos los nombre de las columnas que son de tipo “numerico”. Piense en que el numéro de features podría ser banstante grande.
Respuesta:
## Celda de código para probar.
features_numeric = [feature for feature in df_numeric.columns]
features_numeric
['Calorias',
'Proteina',
'Grasa',
'Sodio',
'Fibra',
'Carbohidratos',
'Azucares',
'Potasio']
Así mismo lo podemos hacer para las no numéricas.
Ejercicio 2.#
Solucione el mismo problema que en el Ejercicio 1 pero con las varibles de tipo “object”.
Respuesta:
## Celda de código para probar.
features_categories = [feature for feature in df_object.columns]
features_categories
['Fabrica', 'Vitaminas']
Matriz de covarianzas y de correlación.#
Cuando se tiene un conjunto de variables cuantitativas, por cada par de ellas se calcula la covarianza:
Estos valores conformarán la matriz de covarianzas. El coeficiente de correlación lineal se define como:
## Matriz de varianzas-covarianzas
df_numeric.cov()
Calorias | Proteina | Grasa | Sodio | Fibra | Carbohidratos | Azucares | Potasio | |
---|---|---|---|---|---|---|---|---|
Calorias | 3895.242099 | 116.442850 | 60.674383 | 4310.041188 | 148.608725 | 416.865952 | 180.380317 | 5362.723526 |
Proteina | 116.442850 | 6.983432 | 1.790252 | 197.706130 | 13.122839 | 12.243296 | 2.850421 | 401.040187 |
Grasa | 60.674383 | 1.790252 | 2.713399 | 55.851817 | 2.284043 | 2.550715 | 3.995474 | 96.005854 |
Sodio | 4310.041188 | 197.706130 | 55.851817 | 17064.098428 | 396.983157 | 468.557877 | 161.021552 | 13109.507474 |
Fibra | 148.608725 | 13.122839 | 2.284043 | 396.983157 | 37.618644 | 10.547819 | 5.329678 | 1065.826587 |
Carbohidratos | 416.865952 | 12.243296 | 2.550715 | 468.557877 | 10.547819 | 71.714955 | -2.017438 | 369.551907 |
Azucares | 180.380317 | 2.850421 | 3.995474 | 161.021552 | 5.329678 | -2.017438 | 34.050018 | 285.976158 |
Potasio | 5362.723526 | 401.040187 | 96.005854 | 13109.507474 | 1065.826587 | 369.551907 | 285.976158 | 32503.973299 |
### Matriz de correlación lineal de pearson
df_numeric.corr().round(4)
Calorias | Proteina | Grasa | Sodio | Fibra | Carbohidratos | Azucares | Potasio | |
---|---|---|---|---|---|---|---|---|
Calorias | 1.0000 | 0.7060 | 0.5902 | 0.5287 | 0.3882 | 0.7887 | 0.4953 | 0.4766 |
Proteina | 0.7060 | 1.0000 | 0.4113 | 0.5727 | 0.8096 | 0.5471 | 0.1848 | 0.8418 |
Grasa | 0.5902 | 0.4113 | 1.0000 | 0.2596 | 0.2261 | 0.1829 | 0.4157 | 0.3233 |
Sodio | 0.5287 | 0.5727 | 0.2596 | 1.0000 | 0.4955 | 0.4236 | 0.2112 | 0.5566 |
Fibra | 0.3882 | 0.8096 | 0.2261 | 0.4955 | 1.0000 | 0.2031 | 0.1489 | 0.9639 |
Carbohidratos | 0.7887 | 0.5471 | 0.1829 | 0.4236 | 0.2031 | 1.0000 | -0.0408 | 0.2420 |
Azucares | 0.4953 | 0.1848 | 0.4157 | 0.2112 | 0.1489 | -0.0408 | 1.0000 | 0.2718 |
Potasio | 0.4766 | 0.8418 | 0.3233 | 0.5566 | 0.9639 | 0.2420 | 0.2718 | 1.0000 |
La matriz anterior muestra la relación lineal entre cada par de variables, por su supuesto en la diagonal principal tenemos la correlación de la variable con ella misma, es decir 1.
Ejercicio 3.#
Haga uso de plotly_express
para construir un scatter plot de las siguientes variables:
Potasio vs. Fibra
## Celda de código para probra.
## Grafico de fibra y potasio.
fig = px.scatter(df_numeric, x = df_numeric.Potasio, y = df_numeric.Fibra)
fig.show()
La relación de las variables anteriores muestra que al parecer a mayor contenido de Potasio se incrementa la fibra.
Ejercicio 4.#
Obtenga el valor de esta correlación haciendo uso de código.
Respuesta:
## Celda de código para probar.
corr = df_numeric.corr().round(4).loc["Fibra","Potasio"]
print("La correlación de la variable Fibra y la variable Potasio es {:.3f}".format(corr))
La correlación de la variable Fibra y la variable Potasio es 0.964
Recuerde que muchas veces un plot dice más que mil palabras… Veamos la matriz de correlación con un mapa de calor
## Mapa de calor de la matriz de correlación.
plt.figure(figsize=(15,6))
sns.heatmap(df_numeric.corr(), annot=True)
plt.show()
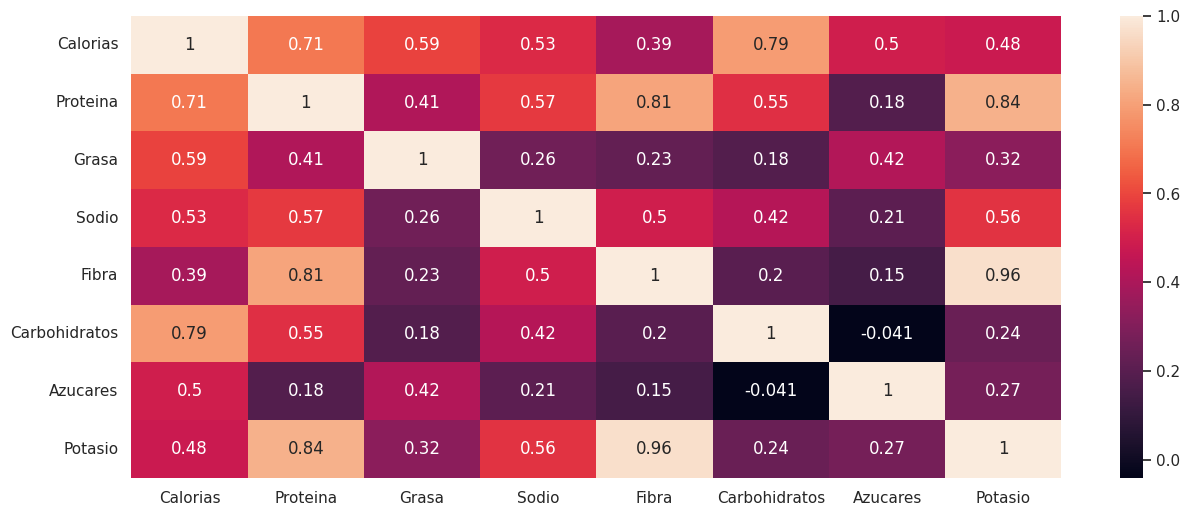
Diagramas de dispersión#
El diagrama de dispersión sirve para visualizar relaciones entre un par de variables cuantitativas.
Ejercicio 5.#
Construya un pairplot
de todas las variables numéricas en el conjunto de datos, y la diagonal presente un kde-plot.
Respuesta:
## Celda de código para probar.
g = sns.pairplot(df_numeric, palette="dark", diag_kind="kde", plot_kws={"color":"red"})
g.fig.set_size_inches(10,10)
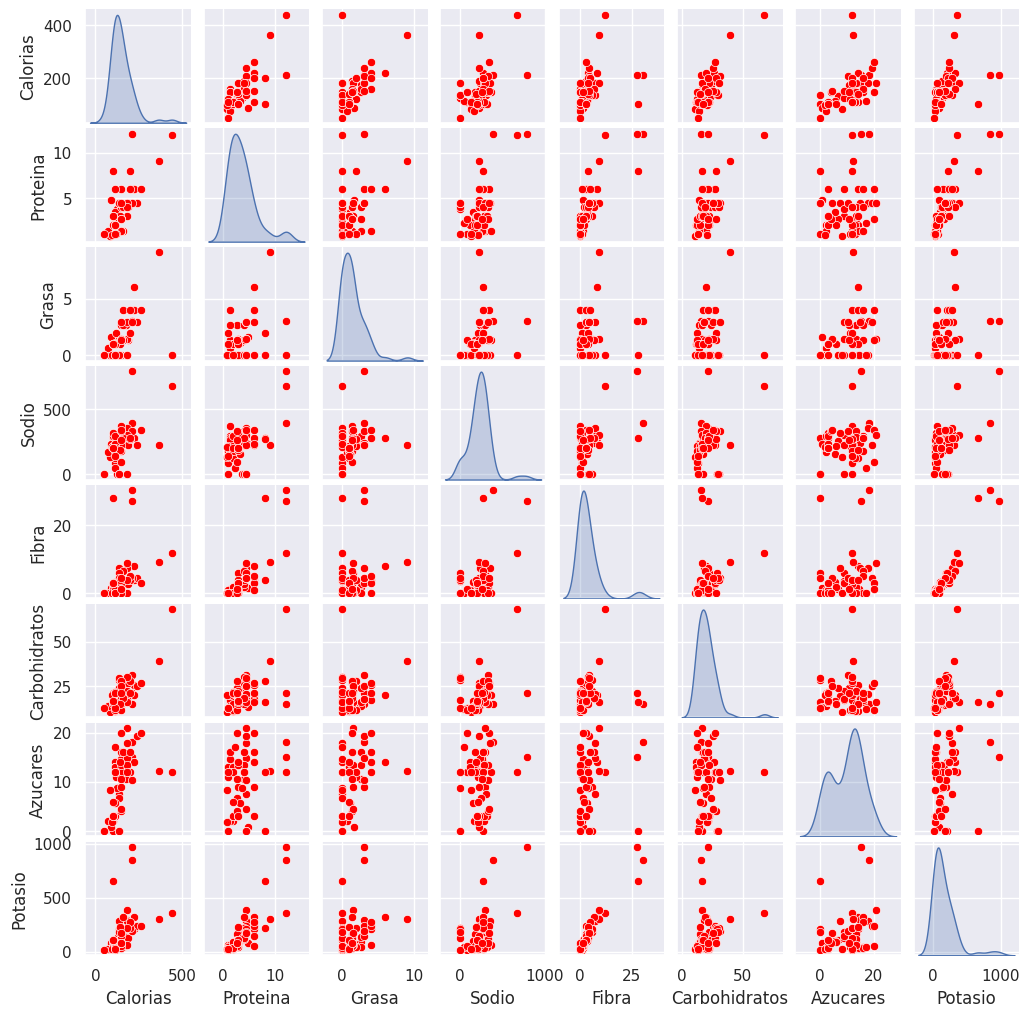
Un plot más dinámico incluyendo el nombre del cereal lo podemos ver con el siguiente código:
## Scatter plot entre Carbohidratos y Azucares. use hover_name = df.index
## Complete el siguiente código
## fig = px.?????(df, x = ???? , y = ????, ?????=?????)
## ???.show()
Replique los mismos gráficos mostrando un scatter-plot de:
Potasio vs. Sodio
Proteina vs. Fibra
## Celda de código para probar.
fig = px.scatter(df, x = "Potasio", y = "Sodio", hover_name = df.index)
fig.show()
## Celda de código para probar.
fig = px.scatter(df, x = "Proteina", y = "Fibra", hover_name = df.index)
fig.show()
Tablas de contingencia.#
Vamos a hacer uso de pd.crosstab()
para construir una tabla de contingencia, recordando que dicha tabla contiene los conteos de una o más variables cualitativas.
url="https://raw.githubusercontent.com/lacamposm/Metodos_Estadisticos/main/data/PruebaSaber1.csv"
df_icfes1 = pd.read_csv(url, sep=";", encoding="latin1",low_memory=False)
df_icfes1.shape
(10000, 78)
crosstab = pd.crosstab(df_icfes1["FAMI_CUARTOSHOGAR"], df_icfes1["FAMI_NIVEL_SISBEN"])
crosstab
FAMI_NIVEL_SISBEN | Esta clasificada en otro nivel del SISBEN | Estrato 1 | Nivel 1 | Nivel 2 | Nivel 3 | No est clasificada por el SISBEN |
---|---|---|---|---|---|---|
FAMI_CUARTOSHOGAR | ||||||
Cinco | 3 | 0 | 116 | 72 | 7 | 72 |
Cuatro | 13 | 0 | 371 | 299 | 70 | 291 |
Diez o m s | 0 | 0 | 1 | 3 | 1 | 3 |
Dos | 35 | 0 | 1757 | 1059 | 199 | 758 |
Nueve | 0 | 0 | 4 | 2 | 0 | 0 |
Ocho | 0 | 0 | 7 | 5 | 1 | 5 |
Seis | 0 | 0 | 38 | 22 | 4 | 21 |
Siete | 0 | 0 | 11 | 10 | 1 | 6 |
Tres | 47 | 0 | 1475 | 1076 | 276 | 936 |
Una | 0 | 1 | 0 | 0 | 0 | 0 |
Uno | 8 | 0 | 460 | 214 | 43 | 141 |
plt.figure(figsize=(15,6))
sns.heatmap(crosstab, annot=True,fmt=".0f")
plt.show()
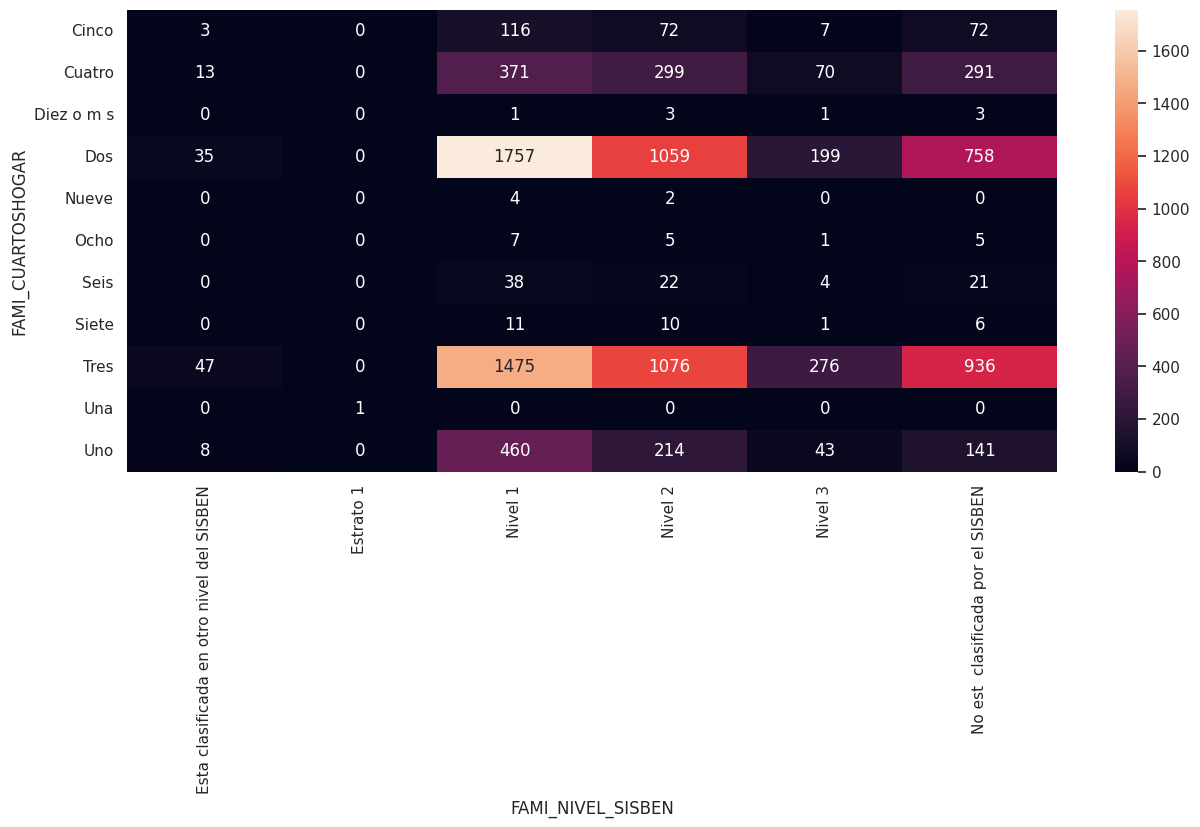
Ejercicio 5.#
¿Que puede comentar del mapa de calor anterior?
Respuesta#
Otros gráficos multivariados.#
df_group = df_icfes1.groupby(["FAMI_CUARTOSHOGAR", "FAMI_NIVEL_SISBEN"]).size()
df_group
FAMI_CUARTOSHOGAR FAMI_NIVEL_SISBEN
Cinco Esta clasificada en otro nivel del SISBEN 3
Nivel 1 116
Nivel 2 72
Nivel 3 7
No est clasificada por el SISBEN 72
Cuatro Esta clasificada en otro nivel del SISBEN 13
Nivel 1 371
Nivel 2 299
Nivel 3 70
No est clasificada por el SISBEN 291
Diez o m s Nivel 1 1
Nivel 2 3
Nivel 3 1
No est clasificada por el SISBEN 3
Dos Esta clasificada en otro nivel del SISBEN 35
Nivel 1 1757
Nivel 2 1059
Nivel 3 199
No est clasificada por el SISBEN 758
Nueve Nivel 1 4
Nivel 2 2
Ocho Nivel 1 7
Nivel 2 5
Nivel 3 1
No est clasificada por el SISBEN 5
Seis Nivel 1 38
Nivel 2 22
Nivel 3 4
No est clasificada por el SISBEN 21
Siete Nivel 1 11
Nivel 2 10
Nivel 3 1
No est clasificada por el SISBEN 6
Tres Esta clasificada en otro nivel del SISBEN 47
Nivel 1 1475
Nivel 2 1076
Nivel 3 276
No est clasificada por el SISBEN 936
Una Estrato 1 1
Uno Esta clasificada en otro nivel del SISBEN 8
Nivel 1 460
Nivel 2 214
Nivel 3 43
No est clasificada por el SISBEN 141
dtype: int64
df_group = df_icfes1.groupby(["FAMI_CUARTOSHOGAR", "FAMI_NIVEL_SISBEN"]).size()
df_group = df_group.reset_index(name="Conteo",)
df_group
FAMI_CUARTOSHOGAR | FAMI_NIVEL_SISBEN | Conteo | |
---|---|---|---|
0 | Cinco | Esta clasificada en otro nivel del SISBEN | 3 |
1 | Cinco | Nivel 1 | 116 |
2 | Cinco | Nivel 2 | 72 |
3 | Cinco | Nivel 3 | 7 |
4 | Cinco | No est clasificada por el SISBEN | 72 |
5 | Cuatro | Esta clasificada en otro nivel del SISBEN | 13 |
6 | Cuatro | Nivel 1 | 371 |
7 | Cuatro | Nivel 2 | 299 |
8 | Cuatro | Nivel 3 | 70 |
9 | Cuatro | No est clasificada por el SISBEN | 291 |
10 | Diez o m s | Nivel 1 | 1 |
11 | Diez o m s | Nivel 2 | 3 |
12 | Diez o m s | Nivel 3 | 1 |
13 | Diez o m s | No est clasificada por el SISBEN | 3 |
14 | Dos | Esta clasificada en otro nivel del SISBEN | 35 |
15 | Dos | Nivel 1 | 1757 |
16 | Dos | Nivel 2 | 1059 |
17 | Dos | Nivel 3 | 199 |
18 | Dos | No est clasificada por el SISBEN | 758 |
19 | Nueve | Nivel 1 | 4 |
20 | Nueve | Nivel 2 | 2 |
21 | Ocho | Nivel 1 | 7 |
22 | Ocho | Nivel 2 | 5 |
23 | Ocho | Nivel 3 | 1 |
24 | Ocho | No est clasificada por el SISBEN | 5 |
25 | Seis | Nivel 1 | 38 |
26 | Seis | Nivel 2 | 22 |
27 | Seis | Nivel 3 | 4 |
28 | Seis | No est clasificada por el SISBEN | 21 |
29 | Siete | Nivel 1 | 11 |
30 | Siete | Nivel 2 | 10 |
31 | Siete | Nivel 3 | 1 |
32 | Siete | No est clasificada por el SISBEN | 6 |
33 | Tres | Esta clasificada en otro nivel del SISBEN | 47 |
34 | Tres | Nivel 1 | 1475 |
35 | Tres | Nivel 2 | 1076 |
36 | Tres | Nivel 3 | 276 |
37 | Tres | No est clasificada por el SISBEN | 936 |
38 | Una | Estrato 1 | 1 |
39 | Uno | Esta clasificada en otro nivel del SISBEN | 8 |
40 | Uno | Nivel 1 | 460 |
41 | Uno | Nivel 2 | 214 |
42 | Uno | Nivel 3 | 43 |
43 | Uno | No est clasificada por el SISBEN | 141 |
fig = px.bar(df_group, x="FAMI_CUARTOSHOGAR", y="Conteo", color="FAMI_NIVEL_SISBEN")
fig.show()
Ejercicio 6.#
¿Observa lo mismo que en el mapa de calor, o quizá se observa información no percibida antes?
Respuesta:
En el repositorio de Github, tenemos más tablas de resultados del ICFES, vamos a importarlas todas y formar un único pandas DataFrame.
Ejercicio 7.#
Construya el pd.DataFrame
solicitado en el párrafo anterior. Llame este DataFrame df_icfes
.
# Celda de código para probar.
url_main = "https://raw.githubusercontent.com/lacamposm/Metodos_Estadisticos/main/data/PruebaSaber"
#
df_icfes = pd.DataFrame()
for i in range(1, 13):
url = url_main + str(i) + ".csv"
df_temp = pd.read_csv(url, sep=";", encoding="latin1", low_memory=False)
print(url," Shape:",df_temp.shape)
df_icfes = pd.concat([df_icfes, df_temp])
print("\nEl tamaño del Dataframe resultante es: {}".format(df_icfes.shape))
https://raw.githubusercontent.com/lacamposm/Metodos_Estadisticos/main/data/PruebaSaber1.csv Shape: (10000, 78)
https://raw.githubusercontent.com/lacamposm/Metodos_Estadisticos/main/data/PruebaSaber2.csv Shape: (10000, 78)
https://raw.githubusercontent.com/lacamposm/Metodos_Estadisticos/main/data/PruebaSaber3.csv Shape: (10000, 78)
https://raw.githubusercontent.com/lacamposm/Metodos_Estadisticos/main/data/PruebaSaber4.csv Shape: (10000, 78)
https://raw.githubusercontent.com/lacamposm/Metodos_Estadisticos/main/data/PruebaSaber5.csv Shape: (10000, 78)
https://raw.githubusercontent.com/lacamposm/Metodos_Estadisticos/main/data/PruebaSaber6.csv Shape: (10000, 78)
https://raw.githubusercontent.com/lacamposm/Metodos_Estadisticos/main/data/PruebaSaber7.csv Shape: (10000, 78)
https://raw.githubusercontent.com/lacamposm/Metodos_Estadisticos/main/data/PruebaSaber8.csv Shape: (10000, 78)
https://raw.githubusercontent.com/lacamposm/Metodos_Estadisticos/main/data/PruebaSaber9.csv Shape: (10000, 78)
https://raw.githubusercontent.com/lacamposm/Metodos_Estadisticos/main/data/PruebaSaber10.csv Shape: (10000, 78)
https://raw.githubusercontent.com/lacamposm/Metodos_Estadisticos/main/data/PruebaSaber11.csv Shape: (10000, 78)
https://raw.githubusercontent.com/lacamposm/Metodos_Estadisticos/main/data/PruebaSaber12.csv Shape: (2504, 78)
El tamaño del Dataframe resultante es: (112504, 78)
corre = df_icfes.select_dtypes(np.number).corr()
plt.figure(figsize=(14, 14), dpi=80)
sns.heatmap(corre,annot=True )
plt.show()
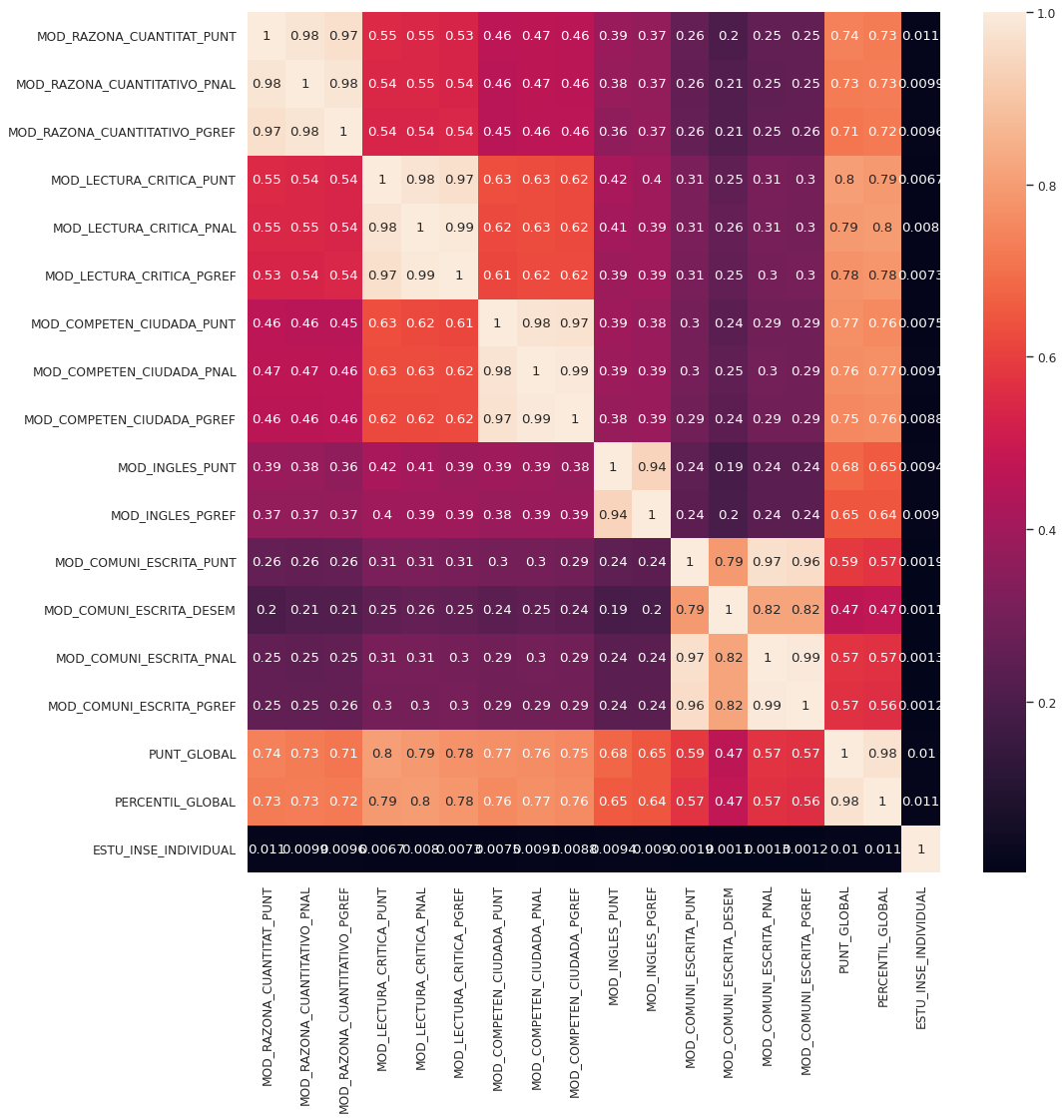
px.scatter(
data_frame=df_icfes,
x="MOD_LECTURA_CRITICA_PUNT",
y="PUNT_GLOBAL",
title="Scatter-plot: Puntaje Lectura crítica y Puntaje global"
).show()
Ejercicio 8.#
Construya con plotly_express
un bar plot del conteo de elementos del cruce entre las variables FAMI_CUARTOSHOGAR
y FAMI_NIVEL_SISBEN
. En el eje \(x\) debe estar la varible FAMI_CUARTOSHOGAR
mientras que en el eje \(y\) el conteo y distinguible por la variable FAMI_NIVEL_SISBEN
.
Hint: Use el parámetro color="FAMI_NIVEL_SISBEN"
df_group1 = df_icfes.groupby(["FAMI_CUARTOSHOGAR", "FAMI_NIVEL_SISBEN"]).size()
df_group1 = df_group1.reset_index(name="Conteo")
px.bar(
df_group1,
x="FAMI_CUARTOSHOGAR",
y="Conteo",
color="FAMI_NIVEL_SISBEN"
).show()
px.scatter(
df_icfes,
x="MOD_RAZONA_CUANTITAT_PUNT",
y="MOD_LECTURA_CRITICA_PUNT"
).show()
Vamos a considerar el DataFrame de las medias para los distintos grupos al cruzar las variables género del estudiante (ESTU_GENERO
) y el departamento (ESTU_INST_DEPARTAMENTO
)
df_group2 = df_icfes.groupby(["ESTU_GENERO", "ESTU_INST_DEPARTAMENTO"])[
df_icfes.select_dtypes(np.number).columns
].mean()
df_group2 = df_group2.reset_index()
df_group2
ESTU_GENERO | ESTU_INST_DEPARTAMENTO | MOD_RAZONA_CUANTITAT_PUNT | MOD_RAZONA_CUANTITATIVO_PNAL | MOD_RAZONA_CUANTITATIVO_PGREF | MOD_LECTURA_CRITICA_PUNT | MOD_LECTURA_CRITICA_PNAL | MOD_LECTURA_CRITICA_PGREF | MOD_COMPETEN_CIUDADA_PUNT | MOD_COMPETEN_CIUDADA_PNAL | MOD_COMPETEN_CIUDADA_PGREF | MOD_INGLES_PUNT | MOD_INGLES_PGREF | MOD_COMUNI_ESCRITA_PUNT | MOD_COMUNI_ESCRITA_DESEM | MOD_COMUNI_ESCRITA_PNAL | MOD_COMUNI_ESCRITA_PGREF | PUNT_GLOBAL | PERCENTIL_GLOBAL | ESTU_INSE_INDIVIDUAL | |
---|---|---|---|---|---|---|---|---|---|---|---|---|---|---|---|---|---|---|---|---|
0 | F | ANTIOQUIA | 101.772414 | 53.034943 | 54.268046 | 105.693333 | 58.797241 | 58.834483 | 103.767356 | 55.794943 | 55.534713 | 102.144368 | 54.313103 | 106.400924 | 2.760739 | 59.833718 | 59.248961 | 103.862529 | 58.614713 | 100.549658 |
1 | F | ATLANTICO | 96.815789 | 45.671053 | 47.680099 | 99.495066 | 49.660362 | 50.739309 | 98.804276 | 48.567434 | 49.110197 | 100.341283 | 53.285362 | 100.752902 | 2.466003 | 51.095357 | 49.945274 | 99.069079 | 48.935033 | 133.363980 |
2 | F | BOGOTA | 96.969663 | 45.931150 | 46.851030 | 99.762009 | 50.002411 | 50.006228 | 100.119699 | 50.410588 | 50.068408 | 97.818383 | 48.282069 | 101.404134 | 2.529434 | 52.469595 | 51.670651 | 99.081668 | 49.123154 | 81.417541 |
3 | F | BOGOTµ, D.C. | 11.000000 | 109.000000 | 66.000000 | 69.000000 | 129.000000 | 92.000000 | 93.000000 | 107.000000 | 62.000000 | 61.000000 | 66.000000 | 68.000000 | 118.000000 | 3.000000 | 82.000000 | 80.000000 | 114.000000 | 84.000000 |
4 | F | BOLIVAR | 95.093143 | 43.630286 | 43.432571 | 99.786857 | 50.230857 | 49.936571 | 98.901714 | 48.574286 | 48.072571 | 100.458286 | 50.823429 | 101.436563 | 2.524221 | 52.294694 | 51.540946 | 98.965143 | 48.772000 | 86.990817 |
5 | F | BOYACA | 96.460432 | 44.863309 | 46.924460 | 100.881295 | 51.410072 | 52.410072 | 102.143885 | 53.428058 | 53.906475 | 92.208633 | 42.607914 | 104.211679 | 2.664234 | 56.029197 | 54.861314 | 98.881295 | 48.568345 | 48.749746 |
6 | F | BUCARAMANGA | 68.000000 | 103.000000 | 55.000000 | 58.000000 | 103.000000 | 57.000000 | 57.000000 | 90.000000 | 31.000000 | 29.000000 | 10.000000 | 10.000000 | 116.000000 | 3.000000 | 79.000000 | 78.000000 | 97.000000 | 44.000000 |
7 | F | CALDAS | 94.621415 | 42.338432 | 47.434034 | 97.403442 | 46.806883 | 52.315488 | 97.296367 | 46.191205 | 51.388145 | 95.642447 | 50.070746 | 103.402724 | 2.626459 | 55.324903 | 59.120623 | 97.311663 | 45.468451 | 45.701468 |
8 | F | CAQUETA | 106.714286 | 59.642857 | 59.500000 | 115.357143 | 68.000000 | 64.000000 | 110.785714 | 61.214286 | 55.785714 | 95.857143 | 44.785714 | 122.857143 | 3.571429 | 84.000000 | 80.071429 | 110.214286 | 68.214286 | 49.708245 |
9 | F | CAUCA | 96.644231 | 45.043269 | 45.197115 | 96.764423 | 45.423077 | 47.317308 | 98.538462 | 47.923077 | 50.307692 | 94.750000 | 45.076923 | 99.497585 | 2.454106 | 49.652174 | 51.903382 | 97.134615 | 45.317308 | 239.199711 |
10 | F | CHOCO | 102.500000 | 53.000000 | 49.000000 | 109.000000 | 66.000000 | 64.500000 | 110.000000 | 63.000000 | 62.500000 | 115.500000 | 75.500000 | 99.500000 | 2.500000 | 49.000000 | 51.500000 | 107.000000 | 62.000000 | 43.185549 |
11 | F | CORDOBA | 90.954545 | 36.909091 | 43.000000 | 93.136364 | 41.363636 | 43.454545 | 92.363636 | 38.772727 | 39.681818 | 88.818182 | 38.590909 | 97.090909 | 2.272727 | 44.000000 | 42.454545 | 92.500000 | 36.181818 | 45.198106 |
12 | F | CUNDINAMARCA | 105.243902 | 58.292683 | 54.829268 | 105.048780 | 57.073171 | 55.975610 | 103.121951 | 53.951220 | 54.000000 | 107.487805 | 58.048780 | 107.780488 | 2.780488 | 61.292683 | 62.682927 | 105.780488 | 62.682927 | 54.448160 |
13 | F | HUILA | 104.855072 | 58.289855 | 58.797101 | 105.086957 | 57.376812 | 58.478261 | 100.782609 | 51.057971 | 52.246377 | 102.927536 | 55.768116 | 108.608696 | 2.855072 | 63.710145 | 64.043478 | 104.507246 | 59.608696 | 47.899190 |
14 | F | LA GUAJIRA | 91.578947 | 39.017544 | 38.596491 | 90.421053 | 38.333333 | 39.877193 | 95.842105 | 44.578947 | 46.456140 | 88.929825 | 34.877193 | 96.910714 | 2.357143 | 46.696429 | 47.446429 | 92.438596 | 37.701754 | 44.642216 |
15 | F | MAGDALENA | 86.131387 | 30.133820 | 38.360097 | 90.416058 | 36.474453 | 40.982968 | 92.469586 | 38.880779 | 42.107056 | 86.520681 | 37.352798 | 96.606516 | 2.303258 | 45.293233 | 46.994987 | 89.863747 | 30.756691 | 44.257546 |
16 | F | META | 103.285714 | 56.000000 | 59.357143 | 104.642857 | 56.571429 | 54.785714 | 106.285714 | 59.000000 | 58.214286 | 103.285714 | 57.571429 | 101.923077 | 2.538462 | 52.153846 | 51.307692 | 102.428571 | 56.000000 | 51.887948 |
17 | F | NARI¥O | 99.586777 | 49.537190 | 53.636364 | 103.157025 | 55.520661 | 56.524793 | 101.123967 | 52.185950 | 52.706612 | 100.995868 | 55.111570 | 105.212500 | 2.695833 | 57.758333 | 57.408333 | 101.859504 | 55.276860 | 47.896739 |
18 | F | NORTE SANTANDER | 96.232353 | 45.014706 | 46.055882 | 96.329412 | 45.247059 | 45.270588 | 96.308824 | 45.238235 | 44.929412 | 92.811765 | 40.808824 | 101.339233 | 2.551622 | 52.572271 | 51.790560 | 96.538235 | 44.052941 | 141.779597 |
19 | F | PUTUMAYO | 98.586957 | 47.282609 | 46.326087 | 106.130435 | 58.304348 | 58.478261 | 107.086957 | 60.478261 | 61.173913 | 101.760870 | 53.717391 | 104.043478 | 2.608696 | 55.456522 | 56.804348 | 103.456522 | 56.543478 | 43.882931 |
20 | F | QUINDIO | 97.160000 | 46.091429 | 44.971429 | 97.920000 | 47.422857 | 45.314286 | 96.954286 | 45.988571 | 44.971429 | 103.771429 | 52.708571 | 105.988439 | 2.722543 | 59.028902 | 57.462428 | 100.148571 | 51.062857 | 55.444022 |
21 | F | RISARALDA | 102.338323 | 52.892216 | 53.224551 | 103.574850 | 54.964072 | 56.476048 | 103.188623 | 54.982036 | 56.697605 | 104.236527 | 58.479042 | 106.639640 | 2.774775 | 60.354354 | 62.684685 | 103.931138 | 57.278443 | 48.747086 |
22 | F | SAN ANDRES | 85.200000 | 28.300000 | 28.200000 | 97.900000 | 46.500000 | 47.000000 | 88.900000 | 35.300000 | 34.900000 | 121.700000 | 78.900000 | 102.888889 | 2.666667 | 56.222222 | 54.333333 | 97.300000 | 45.700000 | 48.792491 |
23 | F | SANTANDER | 102.139052 | 53.400639 | 53.045285 | 103.254129 | 54.831113 | 54.510922 | 102.790623 | 54.378796 | 53.924347 | 100.028237 | 50.905701 | 105.067878 | 2.699091 | 57.965794 | 57.605024 | 102.587640 | 55.923282 | 129.327564 |
24 | F | SUCRE | 84.000000 | 27.391304 | 31.956522 | 87.000000 | 31.782609 | 33.347826 | 87.739130 | 32.695652 | 33.391304 | 89.043478 | 39.260870 | 100.217391 | 2.521739 | 49.521739 | 49.043478 | 89.695652 | 28.869565 | 46.286020 |
25 | F | TOLIMA | 94.158831 | 41.922490 | 43.796696 | 96.062262 | 44.510801 | 46.048285 | 95.589581 | 44.176620 | 45.425667 | 92.886912 | 42.891995 | 98.982028 | 2.423620 | 49.218228 | 49.729140 | 95.331639 | 41.729352 | 228.120692 |
26 | F | VALLE | 100.744059 | 51.374771 | 52.452599 | 103.414381 | 55.513711 | 54.848930 | 101.316880 | 52.062157 | 51.798165 | 101.828763 | 52.672171 | 103.037585 | 2.617991 | 54.805299 | 54.018553 | 101.850701 | 54.382084 | 87.222468 |
27 | M | ANTIOQUIA | 109.816806 | 63.935804 | 62.572025 | 105.901879 | 58.693633 | 57.732777 | 104.268267 | 56.628392 | 56.127349 | 108.071503 | 58.626827 | 103.209424 | 2.604712 | 54.762304 | 55.265445 | 106.186326 | 62.670146 | 53.661166 |
28 | M | ATLANTICO | 101.709589 | 52.874886 | 52.311416 | 97.994521 | 47.587215 | 48.160731 | 96.626484 | 45.789954 | 46.510502 | 104.852968 | 57.042009 | 95.529248 | 2.246054 | 44.000000 | 44.220984 | 99.017352 | 49.296804 | 166.467902 |
29 | M | BOGOTA | 103.188834 | 54.833018 | 53.455043 | 99.886828 | 50.117522 | 49.752648 | 100.057469 | 50.483701 | 50.432245 | 101.509288 | 51.739701 | 97.743063 | 2.362631 | 47.108200 | 47.848990 | 100.289468 | 51.416560 | 98.756578 |
30 | M | BOLIVAR | 100.559278 | 51.036082 | 48.998711 | 96.900773 | 45.922036 | 45.236469 | 95.775129 | 44.567010 | 44.285438 | 101.637887 | 50.856959 | 95.801974 | 2.272368 | 44.223684 | 44.676974 | 97.750000 | 46.362758 | 91.415221 |
31 | M | BOYACA | 105.305085 | 58.186441 | 54.740113 | 100.734463 | 51.033898 | 51.141243 | 105.757062 | 58.378531 | 59.079096 | 96.853107 | 47.090395 | 100.171429 | 2.491429 | 50.754286 | 52.805714 | 101.536723 | 53.807910 | 48.400971 |
32 | M | CALDAS | 96.948370 | 46.103261 | 49.956522 | 94.605978 | 42.961957 | 47.573370 | 94.304348 | 42.581522 | 47.331522 | 95.516304 | 47.500000 | 97.754821 | 2.352617 | 46.911846 | 50.856749 | 95.576087 | 42.290761 | 192.208655 |
33 | M | CALI | 76.000000 | 97.000000 | 44.000000 | 47.000000 | 77.000000 | 13.000000 | 12.000000 | 92.000000 | 34.000000 | 33.000000 | 37.000000 | 38.000000 | 98.000000 | 2.000000 | 44.000000 | 41.000000 | 91.000000 | 28.000000 |
34 | M | CAQUETA | 94.000000 | 40.785714 | 38.500000 | 102.000000 | 52.285714 | 48.071429 | 98.642857 | 49.928571 | 46.785714 | 90.642857 | 33.714286 | 99.000000 | 2.357143 | 48.428571 | 44.928571 | 96.928571 | 43.357143 | 48.510087 |
35 | M | CAUCA | 99.751773 | 50.312057 | 48.773050 | 93.858156 | 42.063830 | 42.460993 | 93.588652 | 40.510638 | 41.971631 | 97.659574 | 46.134752 | 92.496403 | 2.172662 | 41.280576 | 42.848921 | 95.205674 | 41.553191 | 46.978423 |
36 | M | CESAR | 93.500000 | 44.000000 | 49.500000 | 90.500000 | 32.500000 | 44.000000 | 103.000000 | 54.500000 | 66.500000 | 98.500000 | 64.500000 | 96.000000 | 2.000000 | 46.000000 | 53.000000 | 96.000000 | 43.000000 | 50.954109 |
37 | M | CHOCO | 78.375000 | 21.000000 | 18.375000 | 74.250000 | 15.875000 | 15.500000 | 88.375000 | 30.125000 | 30.625000 | 89.625000 | 31.750000 | 72.000000 | 1.375000 | 17.500000 | 19.000000 | 80.500000 | 18.250000 | 47.082766 |
38 | M | CORDOBA | 105.916667 | 61.416667 | 68.416667 | 88.833333 | 35.166667 | 37.166667 | 88.833333 | 39.250000 | 40.333333 | 83.416667 | 30.416667 | 90.500000 | 1.833333 | 33.666667 | 31.833333 | 91.500000 | 33.500000 | 45.957908 |
39 | M | CUNDINAMARCA | 91.578740 | 38.652231 | 47.066929 | 89.620735 | 35.943570 | 45.872703 | 87.051181 | 32.517060 | 40.814961 | 96.337270 | 54.036745 | 90.784392 | 2.070106 | 37.916667 | 43.757937 | 90.950131 | 33.461942 | 178.863560 |
40 | M | HUILA | 106.775510 | 60.183673 | 59.775510 | 99.673469 | 49.755102 | 51.000000 | 98.653061 | 49.591837 | 50.775510 | 97.428571 | 50.551020 | 94.958333 | 2.291667 | 43.875000 | 46.208333 | 99.142857 | 49.816327 | 48.534396 |
41 | M | LA GUAJIRA | 97.774194 | 46.129032 | 44.064516 | 88.693548 | 34.032258 | 36.741935 | 88.790323 | 34.806452 | 38.016129 | 89.483871 | 35.645161 | 94.278689 | 2.213115 | 40.836066 | 43.278689 | 91.532258 | 33.870968 | 46.060031 |
42 | M | MAGDALENA | 90.629630 | 37.137566 | 45.312169 | 91.730159 | 38.148148 | 42.820106 | 93.111111 | 40.338624 | 43.783069 | 91.412698 | 44.084656 | 93.304813 | 2.128342 | 39.700535 | 41.112299 | 91.820106 | 34.936508 | 45.326718 |
43 | M | META | 100.666667 | 51.166667 | 48.833333 | 89.833333 | 32.000000 | 24.166667 | 100.166667 | 49.666667 | 44.500000 | 100.000000 | 39.000000 | 86.833333 | 1.500000 | 27.166667 | 26.500000 | 95.500000 | 40.833333 | 58.642623 |
44 | M | NARI¥O | 104.258427 | 56.247191 | 58.544944 | 100.640449 | 50.853933 | 52.415730 | 101.702247 | 51.994382 | 53.668539 | 102.471910 | 56.112360 | 100.610169 | 2.463277 | 50.468927 | 51.124294 | 101.825843 | 54.016854 | 49.799390 |
45 | M | NORTE SANTANDER | 101.960714 | 53.089286 | 50.832143 | 96.964286 | 46.585714 | 45.850000 | 97.789286 | 47.510714 | 47.400000 | 95.164286 | 42.285714 | 96.336957 | 2.300725 | 45.275362 | 46.362319 | 97.353571 | 45.821429 | 46.721598 |
46 | M | PUTUMAYO | 103.218750 | 55.968750 | 53.343750 | 103.093750 | 56.031250 | 55.218750 | 103.968750 | 55.062500 | 54.718750 | 102.625000 | 52.531250 | 102.281250 | 2.531250 | 53.250000 | 55.406250 | 103.062500 | 56.125000 | 41.657901 |
47 | M | QUINDIO | 109.084942 | 63.536680 | 60.888031 | 101.054054 | 51.590734 | 49.463320 | 99.772201 | 50.185328 | 49.478764 | 113.478764 | 63.030888 | 102.540856 | 2.603113 | 53.412451 | 53.311284 | 105.046332 | 59.934363 | 55.628366 |
48 | M | RISARALDA | 110.291566 | 64.356627 | 63.240964 | 104.380723 | 56.260241 | 57.081928 | 102.978313 | 54.407229 | 55.910843 | 111.253012 | 65.255422 | 104.884337 | 2.674699 | 57.159036 | 59.493976 | 106.763855 | 62.901205 | 50.474686 |
49 | M | SAN ANDRES | 86.333333 | 29.333333 | 27.833333 | 86.333333 | 27.833333 | 26.666667 | 82.000000 | 27.500000 | 27.166667 | 104.666667 | 56.000000 | 84.333333 | 1.833333 | 26.833333 | 25.333333 | 88.666667 | 28.666667 | 44.285651 |
50 | M | SANTANDER | 111.823529 | 66.230273 | 64.321377 | 105.875897 | 58.461263 | 57.911765 | 104.651363 | 56.788379 | 56.700143 | 105.660689 | 57.417504 | 102.475126 | 2.560923 | 53.693583 | 54.702956 | 105.982783 | 62.153515 | 92.774122 |
51 | M | SUCRE | 91.000000 | 37.191358 | 43.185185 | 90.253086 | 36.623457 | 44.345679 | 93.487654 | 41.339506 | 47.759259 | 93.851852 | 48.500000 | 94.277778 | 2.234568 | 42.728395 | 48.135802 | 92.561728 | 35.740741 | 47.704915 |
52 | M | TOLIMA | 101.195596 | 51.818653 | 49.582902 | 96.695596 | 45.715026 | 46.387306 | 94.814767 | 42.713731 | 43.971503 | 96.825130 | 45.930052 | 95.228162 | 2.242503 | 43.242503 | 44.633638 | 96.821244 | 44.252591 | 116.244638 |
53 | M | VALLE | 106.048924 | 58.889106 | 58.080235 | 102.902153 | 54.322244 | 53.395303 | 100.696021 | 51.896934 | 51.884540 | 106.052838 | 56.636660 | 97.157548 | 2.331575 | 46.121292 | 46.602505 | 102.386171 | 55.390085 | 91.522634 |
El siguiente plot, por tanto, muestra un scatter-plot de las medias de 2 variables, separadas por género donde además podemos evidenciar de que departamento se trata.
px.scatter(
df_group2,
x="MOD_RAZONA_CUANTITAT_PUNT",
y="MOD_LECTURA_CRITICA_PUNT",
color="ESTU_GENERO",
hover_name="ESTU_INST_DEPARTAMENTO"
).show()
Ejercicio 9.#
Construya un scatter-plot con plotly_express
de los promedios de las varibles MOD_RAZONA_CUANTITAT_PUNT
, MOD_LECTURA_CRITICA_PUNT
con parámetro size
igual a la cantidad de elementos en los grupos formados por el par de variables cualitativas ESTU_GENERO
y ESTU_INST_DEPARTAMENTO
, separe este plot por el género.
## Celda de código para probar.
df_group3 = df_icfes.groupby(by = ["ESTU_GENERO","ESTU_INST_DEPARTAMENTO"]).size()
df_group3 = df_group3.reset_index(name="Conteo")
df_group2["Conteo"] = df_group3["Conteo"]
##
fig = px.scatter(df_group2, x="MOD_RAZONA_CUANTITAT_PUNT", y="MOD_LECTURA_CRITICA_PUNT",
color="ESTU_GENERO", size="Conteo",
hover_name="ESTU_INST_DEPARTAMENTO")
fig.show()
Finalmente unos gráficos más.
fig = px.density_contour(df_icfes, x = "MOD_LECTURA_CRITICA_PUNT", y = "MOD_RAZONA_CUANTITAT_PUNT")
fig.update_traces(contours_coloring="fill", contours_showlabels = True)
fig.show()
fig = px.density_contour(df_icfes, x = "MOD_RAZONA_CUANTITAT_PUNT", y = "MOD_COMPETEN_CIUDADA_PUNT")
fig.update_traces(contours_coloring="fill", contours_showlabels = True)
fig.show()
fig = px.bar_polar(df_group2, r = "MOD_COMUNI_ESCRITA_PUNT", theta = "ESTU_INST_DEPARTAMENTO",
color = "ESTU_GENERO", template="plotly_dark",
color_discrete_sequence = px.colors.sequential.Plasma_r)
fig.show()
tabla2=df_group2[df_group2["Conteo"]>2]
fig = px.bar_polar(tabla2, r=df_group2.MOD_COMUNI_ESCRITA_PUNT, theta=df_group2.ESTU_INST_DEPARTAMENTO,
color=df_group2.ESTU_GENERO, template="plotly_dark",
color_discrete_sequence= px.colors.sequential.Plasma_r)
fig.show()